Claude 3.7 Sonnet and Claude Code
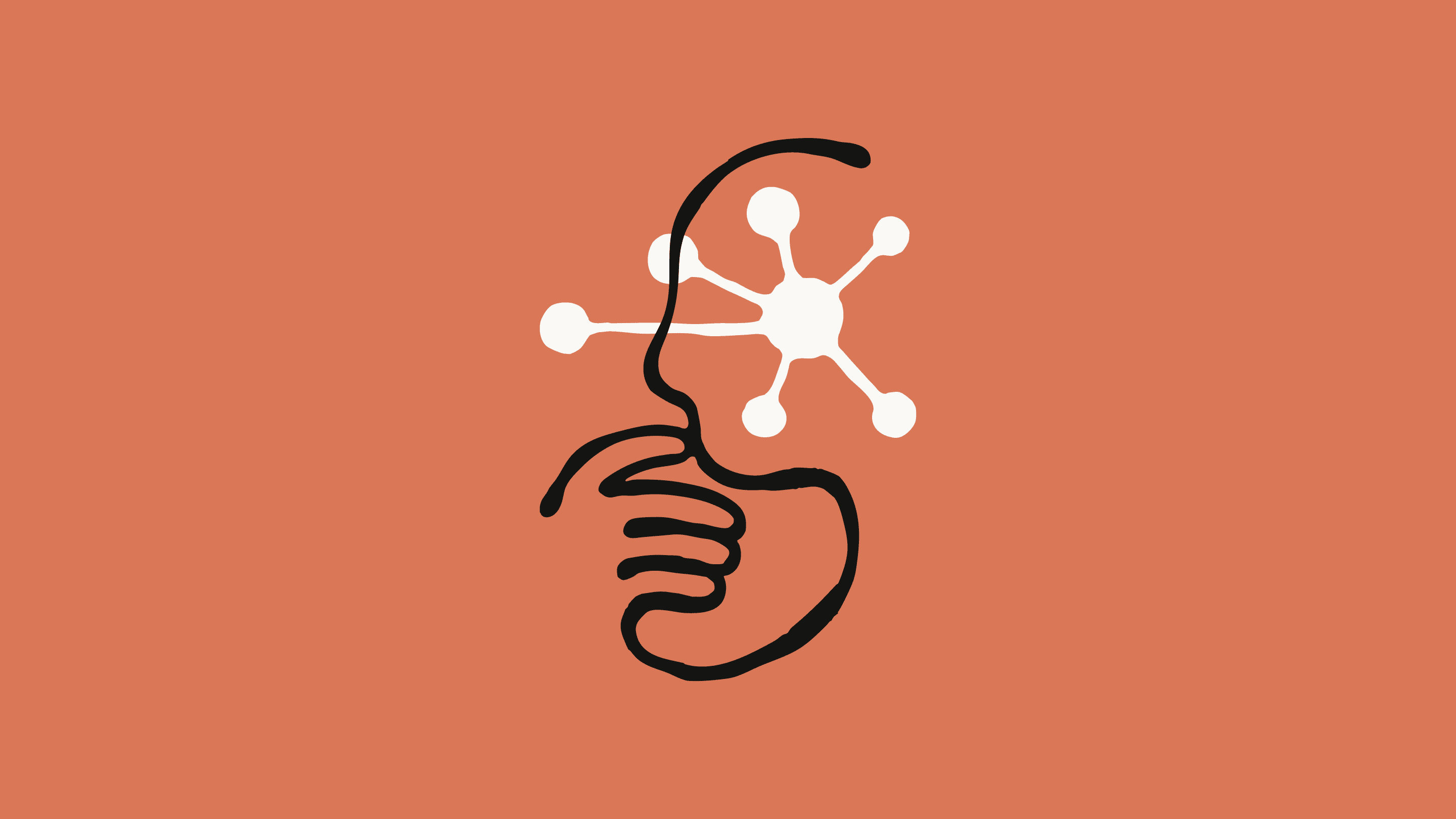
Today, we’re announcing Claude 3.7 Sonnet1, our most intelligent model to date and the first hybrid reasoning model on the market. Claude 3.7 Sonnet can produce near-instant responses or extended, step-by-step thinking that is made visible to the user. API users also have fine-grained control over how long the model can think for.
Claude 3.7 Sonnet shows particularly strong improvements in coding and front-end web development. Along with the model, we’re also introducing a command line tool for agentic coding, Claude Code. Claude Code is available as a limited research preview, and enables developers to delegate substantial engineering tasks to Claude directly from their terminal.
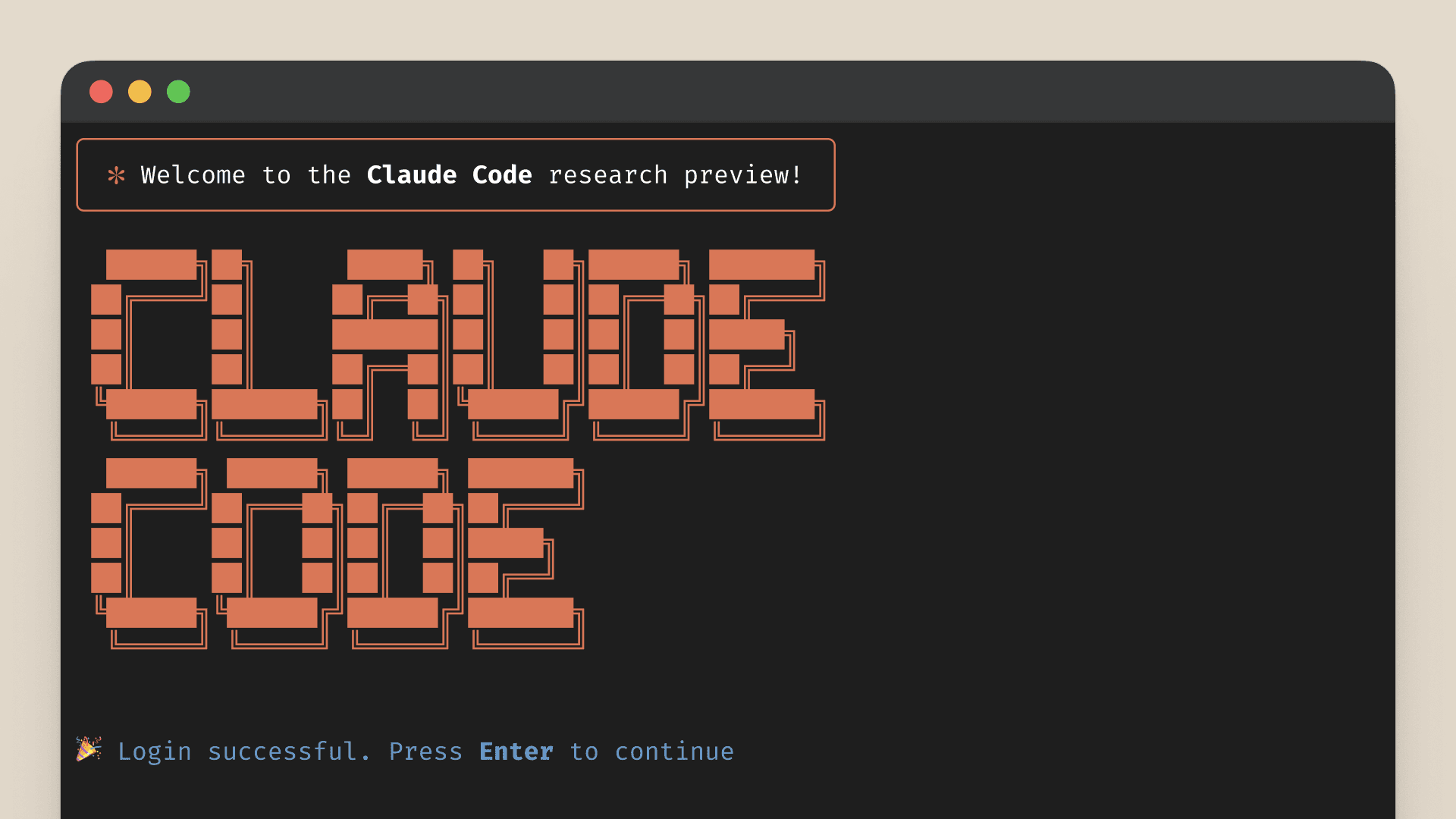
Claude 3.7 Sonnet is now available on all Claude plans—including Free, Pro, Team, and Enterprise—as well as the Anthropic API, Amazon Bedrock, and Google Cloud’s Vertex AI. Extended thinking mode is available on all surfaces except the free Claude tier.
In both standard and extended thinking modes, Claude 3.7 Sonnet has the same price as its predecessors: $3 per million input tokens and $15 per million output tokens—which includes thinking tokens.
Claude 3.7 Sonnet: Frontier reasoning made practical
We’ve developed Claude 3.7 Sonnet with a different philosophy from other reasoning models on the market. Just as humans use a single brain for both quick responses and deep reflection, we believe reasoning should be an integrated capability of frontier models rather than a separate model entirely. This unified approach also creates a more seamless experience for users.
Claude 3.7 Sonnet embodies this philosophy in several ways. First, Claude 3.7 Sonnet is both an ordinary LLM and a reasoning model in one: you can pick when you want the model to answer normally and when you want it to think longer before answering. In the standard mode, Claude 3.7 Sonnet represents an upgraded version of Claude 3.5 Sonnet. In extended thinking mode, it self-reflects before answering, which improves its performance on math, physics, instruction-following, coding, and many other tasks. We generally find that prompting for the model works similarly in both modes.
Second, when using Claude 3.7 Sonnet through the API, users can also control the budget for thinking: you can tell Claude to think for no more than N tokens, for any value of N up to its output limit of 128K tokens. This allows you to trade off speed (and cost) for quality of answer.
Third, in developing our reasoning models, we’ve optimized somewhat less for math and computer science competition problems, and instead shifted focus towards real-world tasks that better reflect how businesses actually use LLMs.
Early testing demonstrated Claude’s leadership in coding capabilities across the board: Cursor noted Claude is once again best-in-class for real-world coding tasks, with significant improvements in areas ranging from handling complex codebases to advanced tool use. Cognition found it far better than any other model at planning code changes and handling full-stack updates. Vercel highlighted Claude’s exceptional precision for complex agent workflows, while Replit has successfully deployed Claude to build sophisticated web apps and dashboards from scratch, where other models stall. In Canva’s evaluations, Claude consistently produced production-ready code with superior design taste and drastically reduced errors.
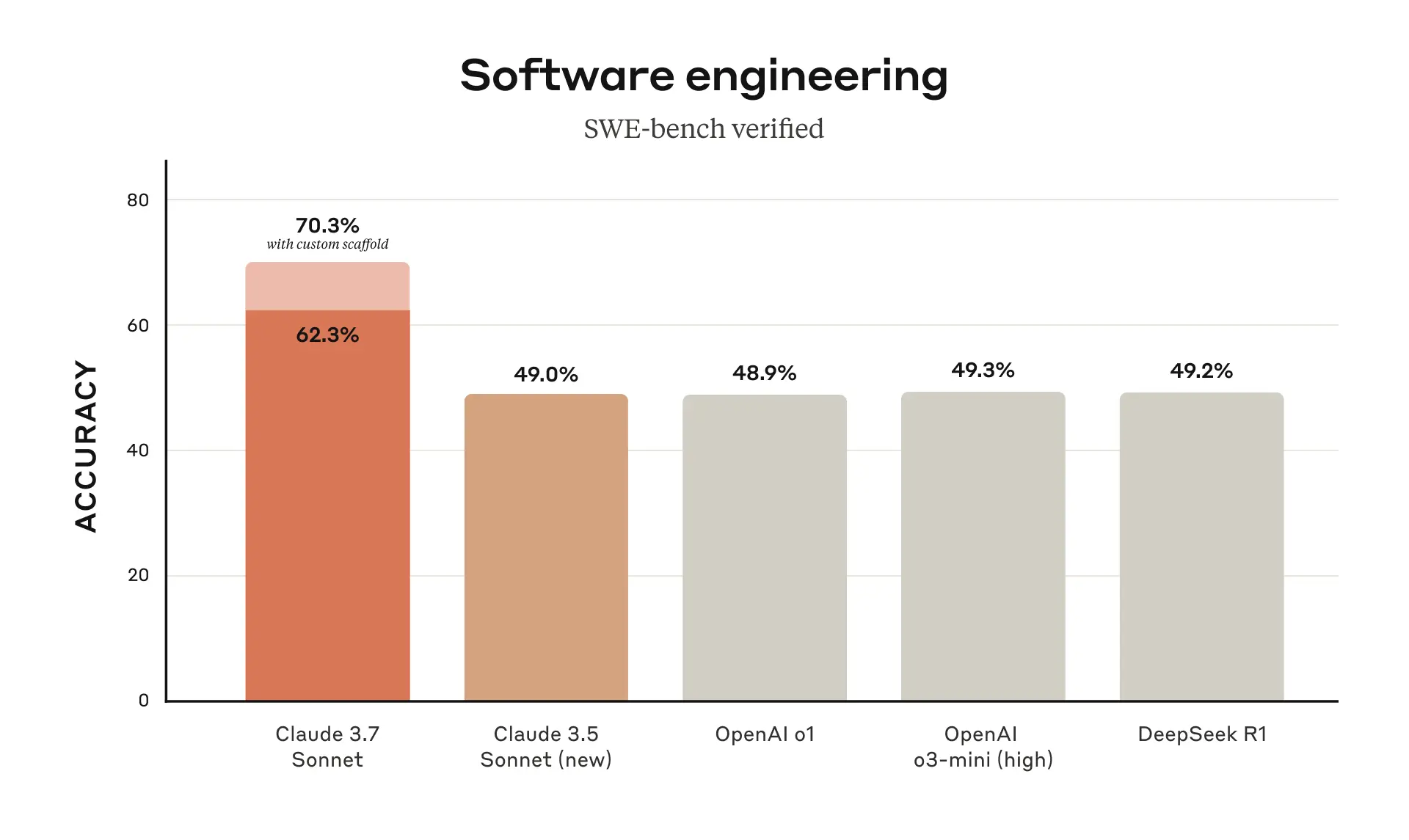
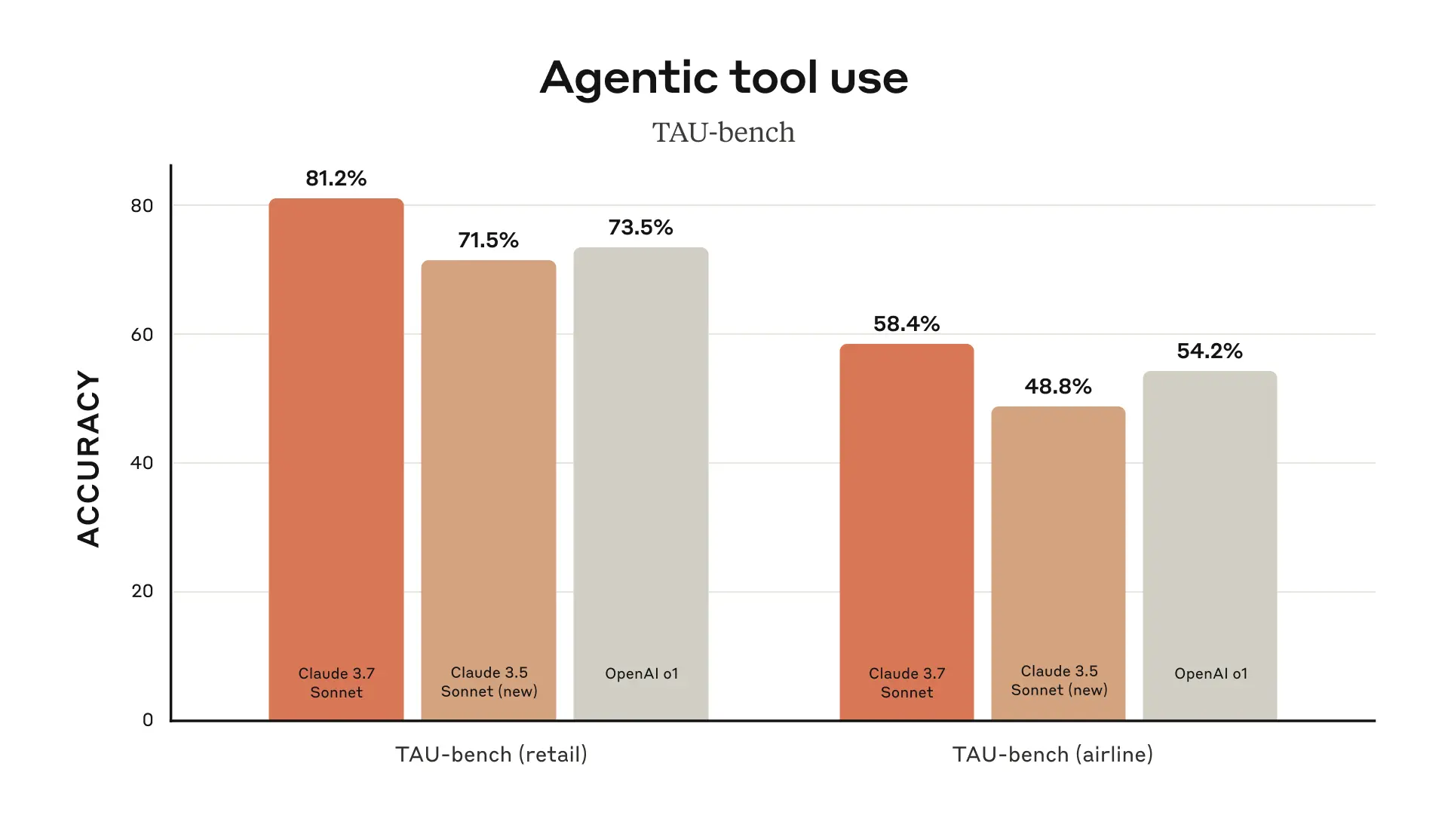
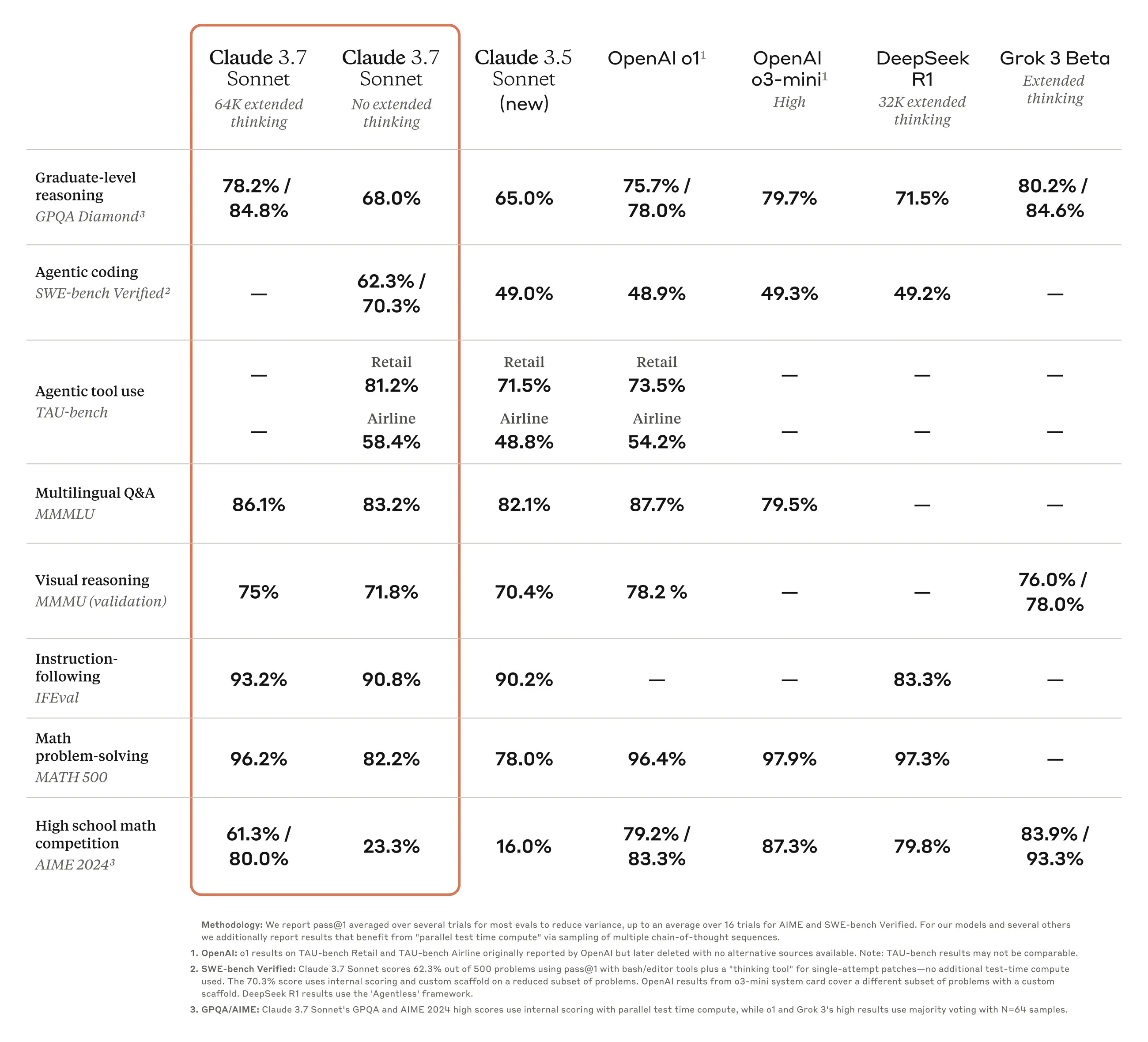
Claude Code
Since June 2024, Sonnet has been the preferred model for developers worldwide. Today, we're empowering developers further by introducing Claude Code—our first agentic coding tool—in a limited research preview.
Claude Code is an active collaborator that can search and read code, edit files, write and run tests, commit and push code to GitHub, and use command line tools—keeping you in the loop at every step.
Claude Code is an early product but has already become indispensable for our team, especially for test-driven development, debugging complex issues, and large-scale refactoring. In early testing, Claude Code completed tasks in a single pass that would normally take 45+ minutes of manual work, reducing development time and overhead.
In the coming weeks, we plan to continually improve it based on our usage: enhancing tool call reliability, adding support for long-running commands, improved in-app rendering, and expanding Claude's own understanding of its capabilities.
Our goal with Claude Code is to better understand how developers use Claude for coding to inform future model improvements. By joining this preview, you’ll get access to the same powerful tools we use to build and improve Claude, and your feedback will directly shape its future.
Working with Claude on your codebase
We’ve also improved the coding experience on Claude.ai. Our GitHub integration is now available on all Claude plans—enabling developers to connect their code repositories directly to Claude.
Claude 3.7 Sonnet is our best coding model to date. With a deeper understanding of your personal, work, and open source projects, it becomes a more powerful partner for fixing bugs, developing features, and building documentation across your most important GitHub projects.
Building responsibly
We’ve conducted extensive testing and evaluation of Claude 3.7 Sonnet, working with external experts to ensure it meets our standards for security, safety, and reliability. Claude 3.7 Sonnet also makes more nuanced distinctions between harmful and benign requests, reducing unnecessary refusals by 45% compared to its predecessor.
The system card for this release covers new safety results in several categories, providing a detailed breakdown of our Responsible Scaling Policy evaluations that other AI labs and researchers can apply to their work. The card also addresses emerging risks that come with computer use, particularly prompt injection attacks, and explains how we evaluate these vulnerabilities and train Claude to resist and mitigate them. Additionally, it examines potential safety benefits from reasoning models: the ability to understand how models make decisions, and whether model reasoning is genuinely trustworthy and reliable. Read the full system card to learn more.
Looking ahead
Claude 3.7 Sonnet and Claude Code mark an important step towards AI systems that can truly augment human capabilities. With their ability to reason deeply, work autonomously, and collaborate effectively, they bring us closer to a future where AI enriches and expands what humans can achieve.
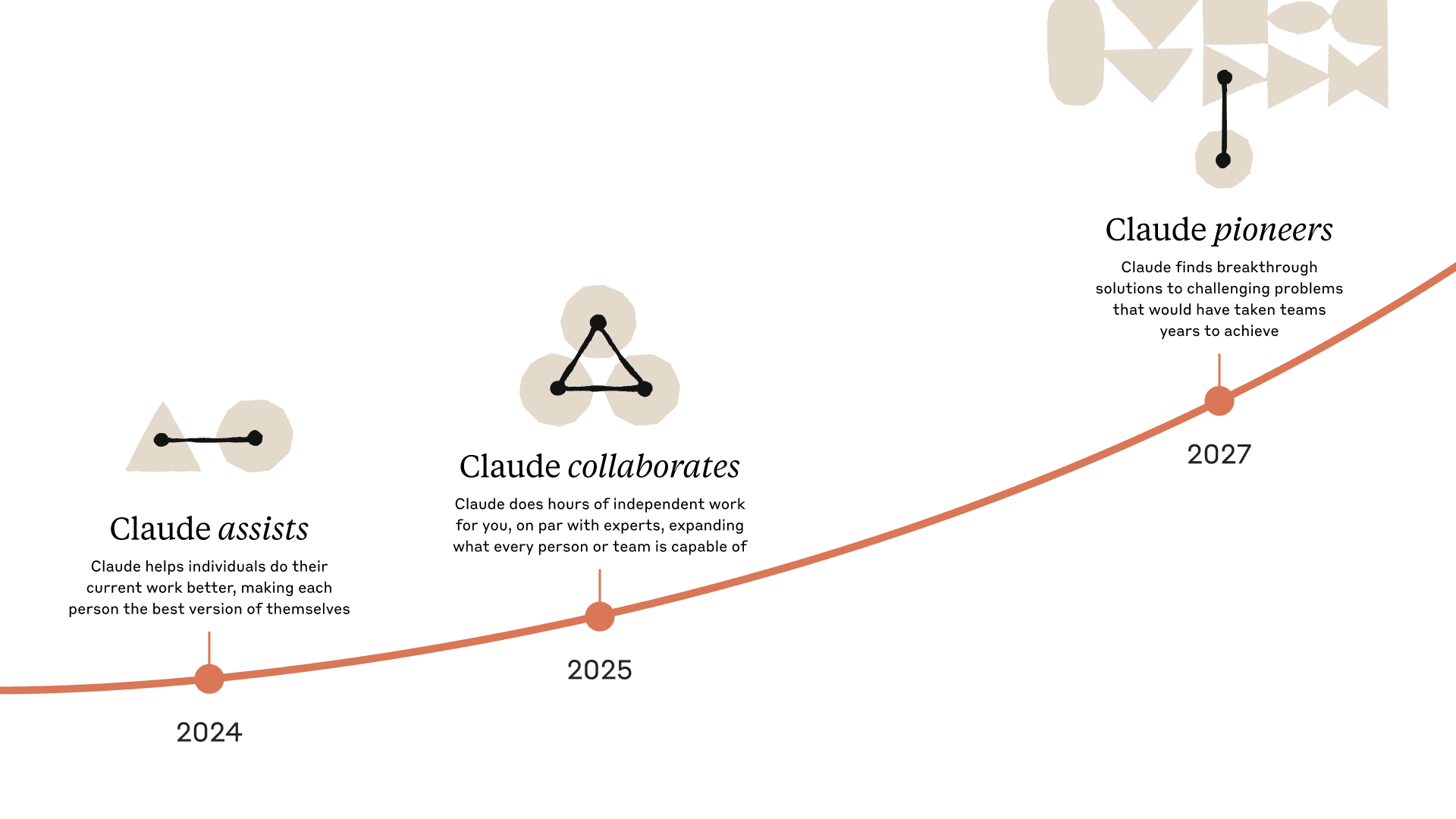
We're excited for you to explore these new capabilities and to see what you’ll create with them. As always, we welcome your feedback as we continue to improve and evolve our models.
Appendix
1 Lesson learned on naming.
Eval data sources
TAU-bench
Information about the scaffolding
Scores were achieved with a prompt addendum to the Airline Agent Policy instructing Claude to better utilize a “planning” tool, where the model is encouraged to write down its thoughts as it solves the problem distinct from our usual thinking mode, during the multi-turn trajectories to best leverage its reasoning abilities. To accommodate the additional steps Claude incurs by utilizing more thinking, the maximum number of steps (counted by model completions) was increased from 30 to 100 (most trajectories completed under 30 steps with only one trajectory reaching above 50 steps).
Additionally, the TAU-bench score for Claude 3.5 Sonnet (new) differs from what we originally reported on release because of small dataset improvements introduced since then. We re-ran on the updated dataset for more accurate comparison with Claude 3.7 Sonnet.
SWE-bench Verified
Information about the scaffolding
There are many approaches to solving open ended agentic tasks like SWE-bench. Some approaches offload much of the complexity of deciding which files to investigate or edit and which tests to run to more traditional software, leaving the core language model to generate code in predefined places, or select from a more limited set of actions. Agentless (Xia et al., 2024) is a popular framework used in the evaluation of Deepseek’s R1 and other models which augments an agent with prompt- and embedding-based file retrieval mechanisms, patch localization, and best-of-40 rejection sampling against regression tests. Other scaffolds (e.g. Aide) further supplement models with additional test-time compute in the form of retries, best-of-N, or Monte Carlo Tree Search (MCTS).
For Claude 3.7 Sonnet and Claude 3.5 Sonnet (new), we use a much simpler approach with minimal scaffolding, where the model decides which commands to run and files to edit in a single session. Our main “no extended thinking” pass@1 result simply equips the model with the two tools described here—a bash tool, and a file editing tool that operates via string replacements—as well as the “planning tool” mentioned above in our TAU-bench results. Due to infrastructure limitations, only 489/500 problems are actually solvable on our internal infrastructure (i.e., the golden solution passes the tests). For our vanilla pass@1 score we are counting the 11 unsolvable problems as failures to maintain parity with the official leaderboard. For transparency, we separately release the test cases that did not work on our infrastructure.
For our “high compute” number we adopt additional complexity and parallel test-time compute as follows:
- We sample multiple parallel attempts with the scaffold above
- We discard patches that break the visible regression tests in the repository, similar to the rejection sampling approach adopted by Agentless; note no hidden test information is used.
- We then rank the remaining attempts with a scoring model similar to our results on GPQA and AIME described in our research post and choose the best one for the submission.
This results in a score of 70.3% on the subset of n=489 verified tasks which work on our infrastructure. Without this scaffold, Claude 3.7 Sonnet achieves 63.7% on SWE-bench Verified using this same subset. The excluded 11 test cases that were incompatible with our internal infrastructure are:
- scikit-learn__scikit-learn-14710
- django__django-10097
- psf__requests-2317
- sphinx-doc__sphinx-10435
- sphinx-doc__sphinx-7985
- sphinx-doc__sphinx-8475
- matplotlib__matplotlib-20488
- astropy__astropy-8707
- astropy__astropy-8872
- sphinx-doc__sphinx-8595
- sphinx-doc__sphinx-9711