Fine-tuning for Claude 3 Haiku in Amazon Bedrock is now generally available
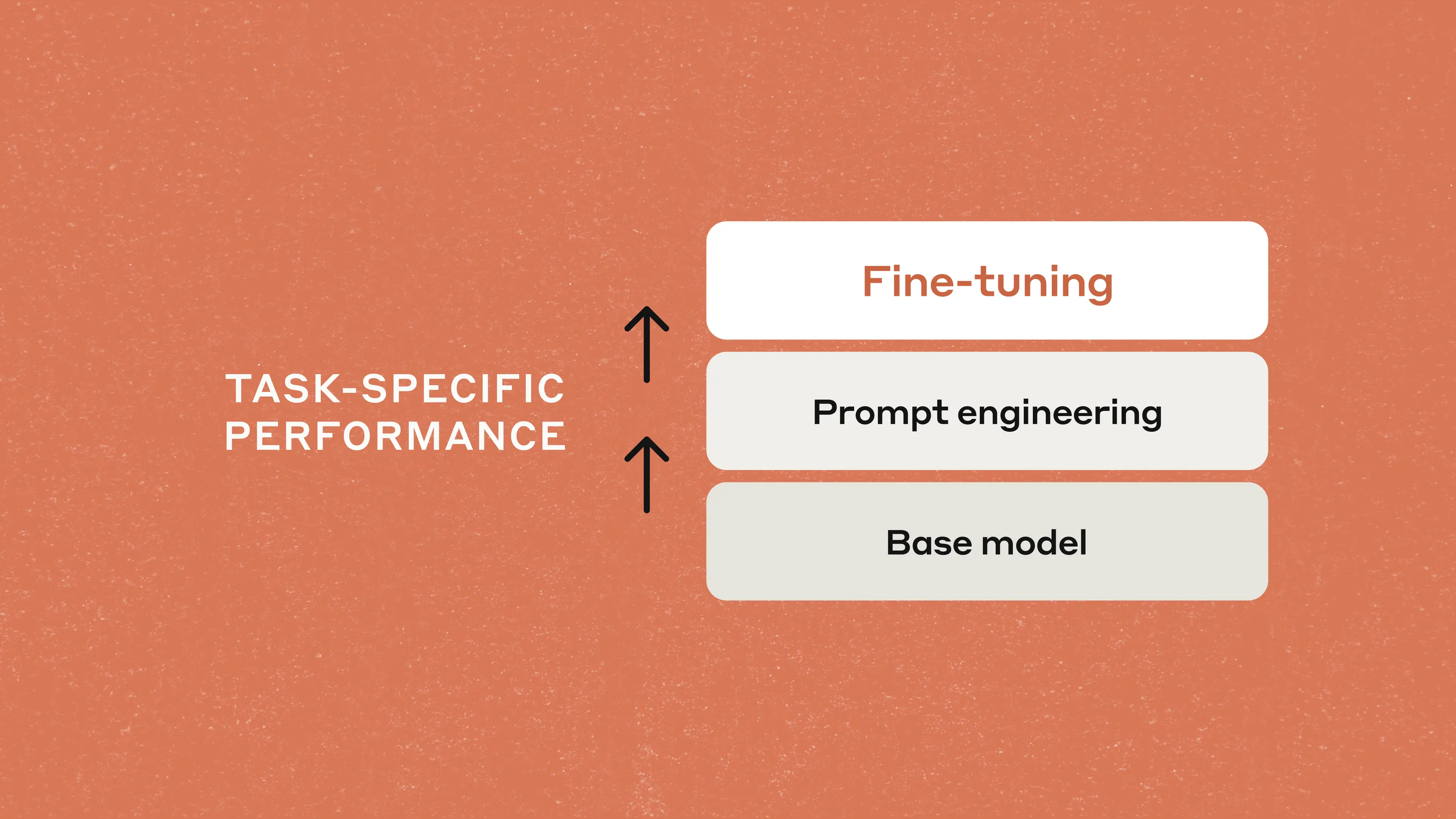
Fine-tuning for Claude 3 Haiku is now generally available in Amazon Bedrock. With fine-tuning, you can customize Claude 3 Haiku—our fastest and most cost-effective model—with your own data to increase model performance for specialized tasks.
Since our preview launch in July, developers have fine-tuned Claude 3 Haiku for use cases ranging from complex legal research analysis to automated financial transaction processing to customer support automation. Now, all Amazon Bedrock users in the US West (Oregon) AWS Region can create, test, and refine their own custom Claude 3 Haiku model via the Amazon Bedrock console and APIs.
Benefits of fine-tuning
Fine-tuning allows you to tailor Claude 3 Haiku’s knowledge and abilities to your use case or industry, making the model more effective for specialized tasks. Benefits include:
- Enhanced domain expertise: Improve accuracy for domain-focused actions like classification and tone and style matching.
- Cost savings and faster response times: Use fine-tuned Claude 3 Haiku in place of Claude 3.5 Sonnet or Claude 3 Opus to reduce costs while increasing speed.
- Simplified deployment: Leverage the Amazon Bedrock API to integrate fine-tuned models into your workflows without extensive AI expertise or the time and resources typically required for developing custom AI solutions.
- Safe and secure: Proprietary training data remains within your AWS environment. Anthropic’s fine-tuning technique preserves the Claude 3 model family’s low risk of harmful outputs.
In partnership with AWS, we fine-tuned Claude 3 Haiku using 10,000 examples from the TAT-QA dataset (which combines tabular and textual financial data) to improve Claude's ability to answer complex questions about financial information. We then tested the fine-tuned model on 3,572 separate examples to assess its performance in financial data analysis and question-answering tasks.
Fine-tuning improved the performance evaluation metric F1 score [1] by 24.6%. Fine-tuned Claude 3 Haiku outperformed the Claude 3.5 Sonnet base model by 9.9%.
Use case | Task type | Metric | Claude 3 Haiku (fine-tuned) | Claude 3 Haiku (base) | Claude 3.5 Sonnet (base) | Improvement versus Claude 3 Haiku (base) | Improvement versus Claude 3.5 Sonnet (base) |
---|---|---|---|---|---|---|---|
TAT-QA | QA on financial text and tabular content | Performance evaluation metric F1 score | 91.2 | 73.2 | 83.0 | +24.6% | +9.9% |
Use cases
Customizing Claude 3 Haiku with your own data improves its performance across a variety of highly specialized tasks. Common use cases include:
- Classification: Fine-tuning can improve Claude 3 Haiku's accuracy in categorizing new data. This is particularly useful for tasks like sentiment analysis, content moderation, or customer support routing.
- Structured outputs: Generate consistently structured outputs tailored to your exact specifications like standardized reports or custom schemas, ensuring compliance with regulatory requirements and internal protocols.
- Brand voice consistency: Fine-tune Claude 3 Haiku to capture your brand's unique tone and vocabulary. This ensures all content–from customer interactions to marketing materials–adheres to your brand guidelines.
Customer spotlight: SK Telecom
SK Telecom, the largest telecommunications company in South Korea, has seen a 75% increase in positive customer feedback, a 40% improvement in key performance indicators, and 35% enhancement in response quality by training a custom Claude model to enable better customer experiences.
"The integration of our fine-tuned large language model into customer support operations has yielded significant improvements in both internal processes and customer satisfaction metrics," shares Eric Davis, Vice President and Head of AI Tech Collaboration Group. “The model's capabilities extend beyond basic interactions, demonstrating proficiency in generating actionable insights from customer call logs, decomposing complex issues into manageable steps, and effectively utilizing integrated tools and APIs. These advancements have streamlined our problem-solving processes and enhanced our ability to address customer needs efficiently.”
Getting started
Fine-tuning for Claude 3 Haiku is now generally available in the US West (Oregon) AWS Region. At launch, we're supporting text-based fine-tuning with plans to introduce vision capabilities in the future.
To get started, log in to the Amazon Bedrock console. Additional details are available in the AWS launch blog, technical deep dive, and documentation.
Footnotes
[1] This example was originally featured in the AWS blog post, “Best practices and lessons for fine-tuning Anthropic’s Claude 3 Haiku in Amazon Bedrock.” F1 Score for LLM evaluation: F1 score is an evaluation metric used to assess the performance of LLMs and traditional ML models.
To compute the F1 Score for LLM evaluation, we need to define precision and recall at the token level. Precision measures the proportion of generated tokens that match the reference tokens, while recall measures the proportion of reference tokens that are captured by the generated tokens. F1 score ranges from 0 to 100, with 100 being the best possible score, and 0 is the lowest. However, interpretation can vary depending on the specific task and requirements.
Precision = (Number of matching tokens in generated text) / (Total number of tokens in generated text) Recall = (Number of matching tokens in generated text) / (Total number of tokens in reference text) F1 = (2 * (Precision * Recall) / (Precision + Recall))*100.
For example : let's say the LLM generates the sentence "The cat sits on the mat in the sun" and the reference sentence is "The cat sits on the soft mat under the warm sun". The precision would be 6/9 (6 matching tokens out of 9 generated tokens), and the recall would be 6/11 (6 matching tokens out of 11 reference tokens).
Precision = 6/9 ≈ 0.667; Recall = 6/11 ≈ 0.545
F1 score = (2 * (0.667 * 0.545) / (0.667 + 0.545))*100 ≈ 59.90